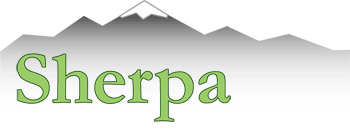
Sherpa: Modeling and Fitting in Python¶
Sherpa is a modeling and fitting application for Python. It contains a powerful language for combining simple models into complex expressions that can be fit to the data using a variety of statistics and optimization methods. It is easily extensible to include user models, statistics and optimization methods.
Sherpa 4.17.1 was released on May 13, 2025. It includes improvements to plotting, several bug fixes, infrastructure and documentation updates.
This version is compatible with Python 3.10 - 3.13. It is available for use outside of CIAO, and can be installed with conda, pip, or built from source. Check the complete 4.17.1 Release Notes and the Sherpa 4.17.1 documentation pages.
Information on recent releases and citation information is available using the Digital Object Identifier (DOI).
Install Sherpa¶
Check How to Install Sherpa? for installation.
There is a set of Notebooks with examples of Sherpa in Python.
See the quick guide to modeling and fitting in Sherpa for a simple introduction to Sherpa’s capabilities.
What can you do with Sherpa?¶
Model generic 1D/2D (N-D) data arrays.
Fit 1D (multiple) data including: spectra, surface brightness profiles, light curves, arrays.
Fit 2D images/surfaces in Poisson/Gaussian regime.
Build complex model expressions.
Import, define and use your own models.
Simulate predicted data based on defined models.
Use appropriate statistics for modeling Poisson or Gaussian data
Use Classic Maximum Likelihood or Bayesian Framework.
Import, define the new statistics, with priors if required by analysis.
Visualize a parameter space with simulations or using 1D/2D cuts of the parameter space
Calculate confidence levels on the best fit model parameters
Use a robust optimization method for the fit: Levenberg-Marquardt, Nelder-Mead Simplex or Monte Carlo/Differential Evolution.
Sherpa supports wcs, responses, psf, convolution.
Use Sherpa as part of astropy.modeling with Sherpa Bridge to Astropy - SABA
Citing Sherpa¶
The new paper describing Sherpa was published in the Astrophysical Journal Supplement Series in 2024. It has been posted on archives.
Please follow the Digital Object Identifier (DOI) <https://doi.org/10.5281/zenodo.593753> for information on how to cite Sherpa.
Release Notes¶
Release Notes for the previous version of Sherpa: